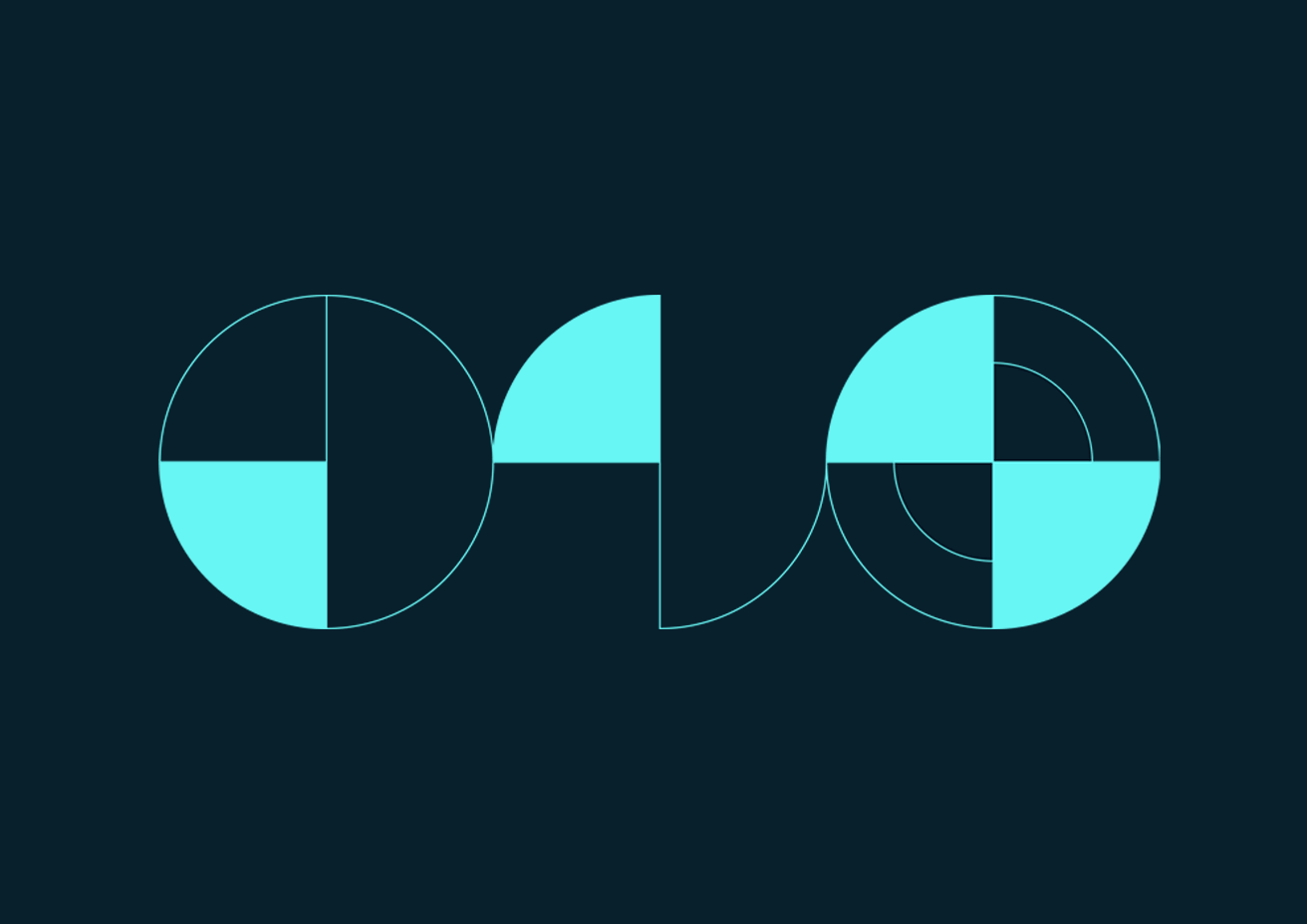
Leveraging Advanced Analytics in Machinery Reliability
How to Tailor Data Analytics to Your Needs and Achieve World-Class Performance
Facilities large and small rely on machinery (rotating equipment) to make their operations tick. Reliable and efficient machinery operation is instrumental in ensuring facilities meet their safety, environmental, and production targets. World-class machinery performance is the result of good design practices, effective and skilled maintenance, and operation within appropriate design conditions (which, depending on operating requirements, can be challenging).
An ever-increasing pool of data and analytic techniques are becoming available to monitor, troubleshoot, and identify improvement opportunities. Rarely are these analyses performed in isolation, as they rely on numerous factors such as process conditions, operating modes, equipment design, and maintenance data. Depending on the quality of data available, identifying and cleansing those data can be a time-consuming and tedious activity. This is especially true if the analysis involves exporting data to a spreadsheet or other external tools.
How Seeq Workbench Makes Data Move Seamlessly
Seeq Workbench offers a powerful suite of advanced analytics tools and add-ons to simplify and speed up complex analyses, and, when combined with Seeq Organizer, those analyses can be seamlessly published and shared with stakeholders across an organization. It does this by natively connecting to a number of data sources and enabling users to identify periods of interest, cleanse data, and apply a variety of algorithms to extract actionable information.
Seeq is designed with the end-user in mind, allowing complete flexibility on what kind of analytics to use. With the recent addition of Seeq Data Lab, users now have the expanded ability to develop advanced analyses leveraging the full Python programming language, libraries, and associated machine learning algorithms. Data moves seamlessly between Workbench, Organizer, and Data Lab, allowing effective collaboration between data scientists, engineers, and subject matter experts.
What are Advanced Analytics?
Seeq’s advanced analytics application leverages innovations in big data, machine learning, and advanced web technologies to integrate and connect to disparate data sources within process manufacturing and drive business improvement.
Users are empowered to take advantage of:
- Diagnostic analytics: Save on unproductive downtime by solving a performance issue and determine its root cause.
- Monitoring and alerts: Easily stay updated on all equipment performance metrics without sifting through unnecessary data.
- Predictive analytics: Identity when maintenance is necessary on equipment before downtime occurs, based on historical data, time-series data, and pattern recognition.
- Repeatable analysis: Easily monitor all performance levels against models of ideal conditions with scalable calculations.
- Data security AND collaboration: Share analyses and data between an entire operation, seamlessly and securely. The technology follows specific data governance protocols to the operation and is easy to manage.
Deploying Advanced Analytics to Empower Machinery Subject Matter Experts
Combining advanced analytics with Seeq’s user-friendly tools to easily cleanse data, filter noise, remove non-operating conditions, and exclude non-value-adding data in the analysis, actionable information can be extracted from a wide range of time-series data without the need to transfer those data into external tools. This accelerates data analysis and empowers quality decision making.
Listed below are several machinery use case examples on how Seeq can be leveraged to drive world-class machinery performance.
Pumps
- Track operating windows vs. OEM curve in near real-time with automated calculations comparing the operating point to Best Efficiency Point (BEP).
- Calculate and compare hydraulic power consumption vs. driver power consumed to detect and address inefficiencies and quantify such losses to help justify upgrades or maintenance intervention.
- Quantify Net Positive Suction Head (NPSH) margin, including in situations where no suction pressure is available by using source vessel pressures, levels, temperatures, and pressure drop calculations to determine suction pressures in near real-time. Correlate low NPSH events with vibration data, aggregate total time spent in low NPSH margin operation, and identify operating strategies to reduce the potential for cavitation.
- Track spare run programs and roll up compliance into automated dashboards.
- Monitor seal flush performance and easily identify changes in key parameters (rising, dropping, or step changes).
- Automatically calculate availability and reliability metrics by combining runtime process data with Computerized Maintenance Management System (CMMS) work order/failure code data.
- Deploy advanced algorithms or machine learning models to identify the onset of a bearing failure in time or frequency domains (if available).
Compressors
- Calculate efficiency/power absorption vs. design and automatically quantify associated energy losses or losses due to other inefficiencies (excessive recycle, etc.).
- Train compressor performance models post overhaul and monitor deviation from this model over time as an early indicator of performance degradation or fouling.
- Monitor auxiliary systems, including automatically identifying changes in seal gas or lube oil pressures, temperatures, and/or filter performance.
- Identify changes to critical parameters such as vibration or primary vent pressures and perform a regression analysis to project into the future when an associated trip limit will be reached.
- Automatically calculate depressurization timing after shutdown to identify potential issues with isolation/blowdown valves or rapid decompression events.
- Collaborate on and publish root cause failure analyses. Leverage advanced algorithms to identify correlation and causation between process and machinery parameters.
Gas Turbines
- Calculate fuel consumption and efficiency in near real-time.
- Detect abnormal increases (or decreases) on inlet air filter dP for a wide range of operating scenarios before alarm thresholds are reached.
- Model emissions based on measured input signals—leverage advanced algorithms using Seeq Data Lab and Python libraries as needed.
- Track running hours on components, calculate remaining component life based on firing temperatures, starts and stops, etc.
- Aggregate operational statistics and process data to optimize or extend overhaul intervals.
Steam Turbines
- Calculate energy consumption and efficiency using Seeq’s built-in steam properties library.
- Track safety system checks such as overspeed trip testing data.
- Monitor steam system parameters and quickly identify excursions that could impact steam quality.
The above is not an exhaustive list; it’s meant to provide ideas on ways advanced analytics with Seeq can help maximize machinery reliability and efficiency in your production facilities. Because Seeq is agile by design, users can design, build, and test analyses customized to organizational priorities. All analyses and reports can be done in one place without the need to export data to spreadsheets. Once in place, the analyses can be applied over a wide time range and scaled across assets as desired.
If your organization relies on reliable and efficient machinery operation, Seeq has tools and technology to help achieve world-class performance while giving end users the flexibility to tailor analyses for their specific needs.
Schedule a time for a demo with one of Seeq’s team members today to see the technology in action!