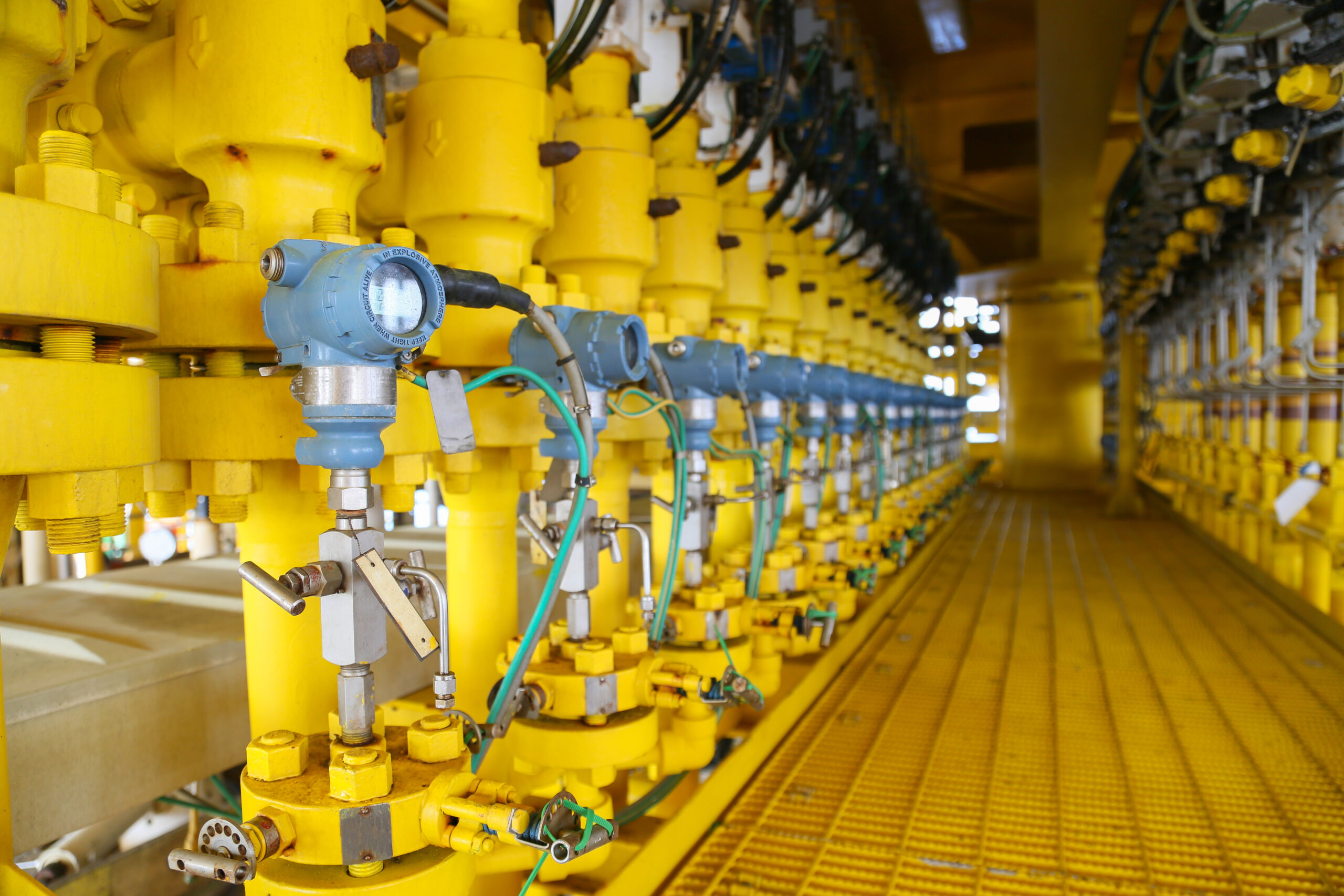
Weather Based Load Predictions
Challenge
The effect of weather variables has long been an under used factor in load forecasting models. Electric load profiles of residential and commercial customers have shown significant correlation between weather & load. Utilities aim to use weather data to predict future demand. Weather properties such as temperature and relative humidity are provided by the weather stations located in a service zone. Because some service territories are large, the use of multiple weather stations allows for better locational forecasting. Combining multiple weather profiles helps the forecasting model explain additional variations of the load spread. Other factors to be considered are the distribution of the load data, the geographic distribution of the service territory, and the location and number of weather stations. All must be considered for the most robust performance and accurate model forecasts.
Solution
- Seeq Cortex is used to integrate NOAA & local weather forecast data and OSIsoft Future Data into a single environment for analysis.
- Seeq’s prediction tool is used to evaluate correlations between load conditions and local weather and create regression models trained on historical data to create continuous load predictions.
- Model error is built into forecast visualizations to show the range of possible outcomes as it extends further out in time.
- These same analytics are applied across multiple geographical locations, customer classes and critical assets using an asset tree built in Seeq.
Results
- Engineers and operators can quickly spot oncoming problems and implement mitigation strategies.
- Successfully identify loads for significant system impact.
- Deferred major capex project for circuit/substation upgrade.
- Leverage DR program for greater success and reliability.
Calculations & Conditions
Seeq’s calculation engine can seamlessly apply analytics across both historical and future data. One major complexity of working with “future” data is the large degree of uncertainty and high propensity to change. Enabling performant calculations on large datasets requires context on which portions of the data set can be categorized as historical data versus future forecasts that are subject to change. Capsules are created for these historical and future states. Models trained on the historical data are created to analyze the load conditions and weather impact and identify areas of concern in future data. Calculations can be scaled across multiple zones or assets leveraging the Treemap view in Seeq Workbench.
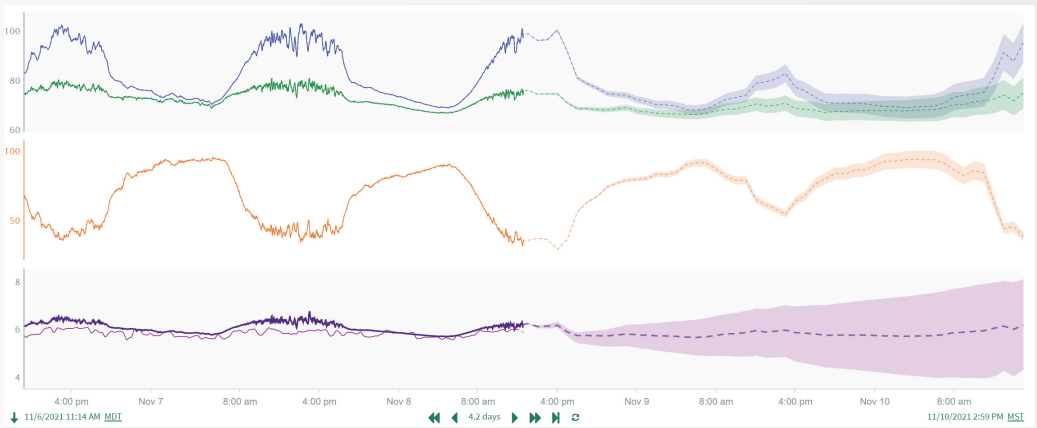