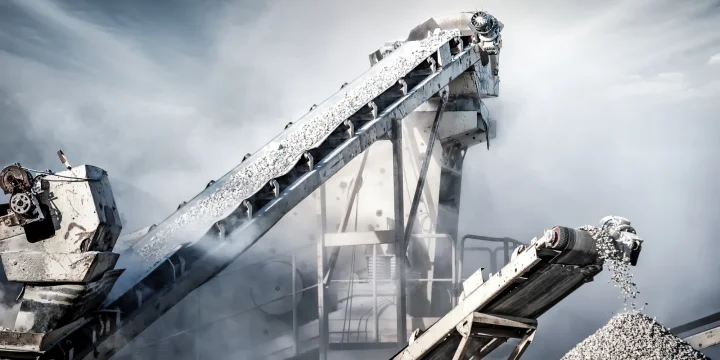
Predict Conveyor Belt Tensioning Date
Challenge
Conveyor belts are a humble yet essential asset for mining companies. They are utilized to transport solids collected from the mines through various processing steps, eventually transferring the materials to a shipping vessel for transport to the final customer. Interruption of conveyor-belt operation threatens the on-time delivery of goods to customers, impacting profitability.
Over time, the tension on a conveyor belt decreases resulting in tracking shifts from side to side. Soon after it begins tracking poorly, it fails. In a system with 100 belts, there would typically be at least one belt failure per day. Historically, it has been difficult to achieve optimal conveyor belt tensioning schedules for a large network of belts. The traditional approach–performing maintenance on a weekly or other periodic basis–often results in unnecessary and costly mechanical activity. Despite the drawbacks to time-based maintenance, companies continue to deploy these strategies because conveyor belt tension behavior is difficult to characterize and predict using existing tools.
A modern approach is to use condition-based monitoring to flag when a belt is due for repairs. Time and a common failure mode profile are often enough information to proactively inform of a belt failure. Identifying and modeling based on this profile has proven challenging with historical tools, but has been made possible through recent developments in advanced analytics. These new technologies are prompting many large mining companies to abandon traditional time-based maintenance approaches in favor of condition-based maintenance schedules predicted by analytical models.
Creating a predictive model for conveyor belt tension enables condition-based maintenance scheduling, reducing unnecessary spending on time-based maintenance and catching unplanned belt failures before a line stoppage event occurs. Calculated conservatively, preventing one belt failure per day could prevent a line stoppage of one hour per day, with a production capacity improvement of 2%.
A global mining company with over 10,000 employees wanted to better predict when its fleet of conveyor belts would require maintenance to re-tension. Seeq advanced analytics software was used to create a predictive model for conveyor belt tension failure, then scale the analysis across the fleet of belts.
Solution
The engineering team for the mining company used Seeq advanced analytics software to create predictive models for conveyor belt tension based on time under tension and historical failure modes. Using Seeq, the team was able to identify the failure date and warning period, scaling analysis to the company’s entire network of conveyor belts.
Seeq capsules identify when a belt begins displaying end of life tension behavior. Treemaps are used to gather a high-level view of which conveyor belts are most in need of maintenance. Each belt’s condition is categorized as red, green, yellow to visually depict which asset is at the highest risk for a tension failure (those marked in red).
The predictive model enables the mining company to shift to condition-based maintenance as opposed to the unappealing options of inefficient scheduled maintenance or simply running to failure. Having visibility into the belts’ future condition enables operators to divert product from a conveyor belt that will fail soon to a different belt flow line.
Using Seeq, the engineers built an asset tree containing the tension signals for all belts, did the necessary analysis one time, and scaled it out to all the belts in the facility, quickly identifying belts with a downward sloping tension profile. Then the team used Seeq’s modeling capabilities to forecast the end-of-life tension behavior into the future, identifying the time when this would fall below an allowable value, enabling proactive corrections to be made and continuous improvements over time.
Results
Using Seeq’s analysis for creating models and scaling them using the Treemap visual, complete with embedded values of time remaining until maintenance, the mining operation was able to quickly and successfully implement a condition-based maintenance program.
Preventing conveyor-belt failures can prevent line back-ups and lost throughput while minimizing scheduled maintenance costs. The mining company is projected to save up to $1 million annually by recouping lost-opportunity cost, as well as saving $100,000 in scheduled and break-in maintenance annually.
Data Sources
- Process Data Historian: OSIsoft PI
- Asset Structure: OSIsoft Asset Framework
Data Cleansing
- Seeq Data Lab is used to build the asset tree structure for scaling the analysis from one asset to the entire fleet.
- Seeq Workbench enables removal of downtimes and signal smoothing to facilitate more resilient models.
Calculations and Conditions
- Use conditions to identify the tension cycles.
- Calculate a baseline belt tension by taking an average belt tension from the left-hand-side and ride-hand-side tension readings.
- Identify when there is a rapid decrease in the tension signal by taking the derivative.
- Create a projection model of the belt tension training the model over the time period in which a failure mode has been detected and project the model into the future.
- Identify when the projected belt tension falls beyond the low-tension limit (50% of baseline or otherwise defined by SME) and conservatism factor.
- Calculate the time until failure using Seeq’s Signal from Condition.
- Scale across dozens of conveyor belts using Treemaps.
Reporting and Collaboration
- Seeq Organizer Topic set to a Live Document to identify maintenance flags in near real-time to inform the Operations Center.