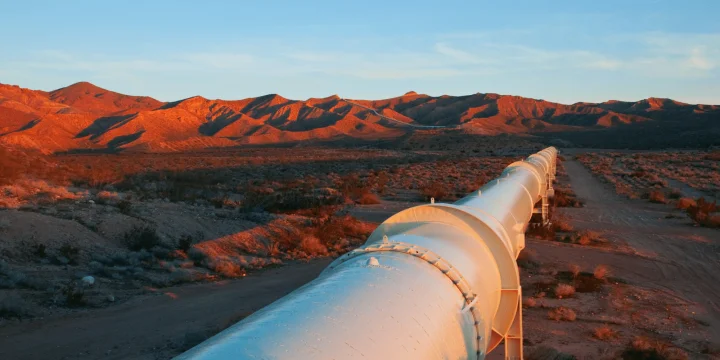
Lockout Pressure Optimization
Challenge
Pipeline operators face a constant balancing act of running their pipelines while protecting the environment from oil spills caused by a rupture. A sudden low-pressure event might indicate a pipeline rupture that threatens the environment. To minimize environmental impact, operators set parameters for low-pressure conditions that would cause a hard shutdown, or lockout. Lockouts can last for hours or days, greatly impacting the pipeline’s revenue.
The pressure sensor is at the discharge side of the pumping station, and in the case of a pipeline rupture, the pressure drops since the internal contents are exposed to the atmosphere. The pressure can drop for reasons other than an oil spill, however. Natural pressure drops can occur due to process variation, causing unnecessary lockouts. The root cause is not always easily understood. Determining the proper pressure at which to shut down the pipeline requires large volumes of data and data cleansing that is difficult, if not impossible, to perform in a spreadsheet. As a result, operators often run at an arbitrary sub-optimal low discharge lockout pressure, resulting in unnecessary lockouts that result in increased startup costs and reduced production uptime.
A major pipeline operator was unable to optimize its operation’s lockdown pressure, as it was too difficult to calculate per station. The operator wanted to avoid unnecessary shutdowns of its pipeline by optimizing its lockout pressure based on operational data rather than arbitrary set points. The goal was to reduce the number of times the system locked itself out by better identifying the pressure at which each pumping station should be locked out.
Solution
Using Seeq, the team examined six months’ worth of data from the operator’s pipeline, doing aggregations of each period of operation for the pipeline. The team identified the following time periods: false data was reported, station was running normally, and transient periods of startup and shutdown. The time periods were filtered to display the pressure signal for times of steady state, normal, pressure. Next, the lowest pressure (or first percentile value) was found during each period of station operation and reported in a tabular view. Finally, using percentile logic, a new minimum discharge pressure was determined from the lowest pressure signal.
The original discharge lockout pressure setting had been 400 psi. After the analysis done in Seeq, the team determined the ideal setting was 308 psi, resulting in fewer lockouts without increasing risk to the environment.
Results
The new, optimized low discharge lockout pressure of 308 psi was calculated based on the initial study period with the ability to continually monitor and finetune pipeline performance efficiently over time. The result of being able to optimize the lockout pressure was a reduction of unnecessary station shutdown events that added up to more than 30 hours per quarter. The associated cost savings were $1.5 million per event, thanks to the avoidance of lost production and station startup time.
Data Sources
- OSIsoft PI
- CSV files
Data Cleansing
- Capsules are used to identify when the station is running; capsule logic is then tuned based on engineering expertise to remove startup and shutdown periods to ensure they do not impact limit calculations.
alculations and Conditions
- Value Search, Formula, Capsule View, Signal from Condition, Scorecard
Reporting and Collaboration
A Topic was created summarizing the goal and initial findings that also incorporated quarterly monitoring of the metrics created to further finetune the low discharge lockout pressure.