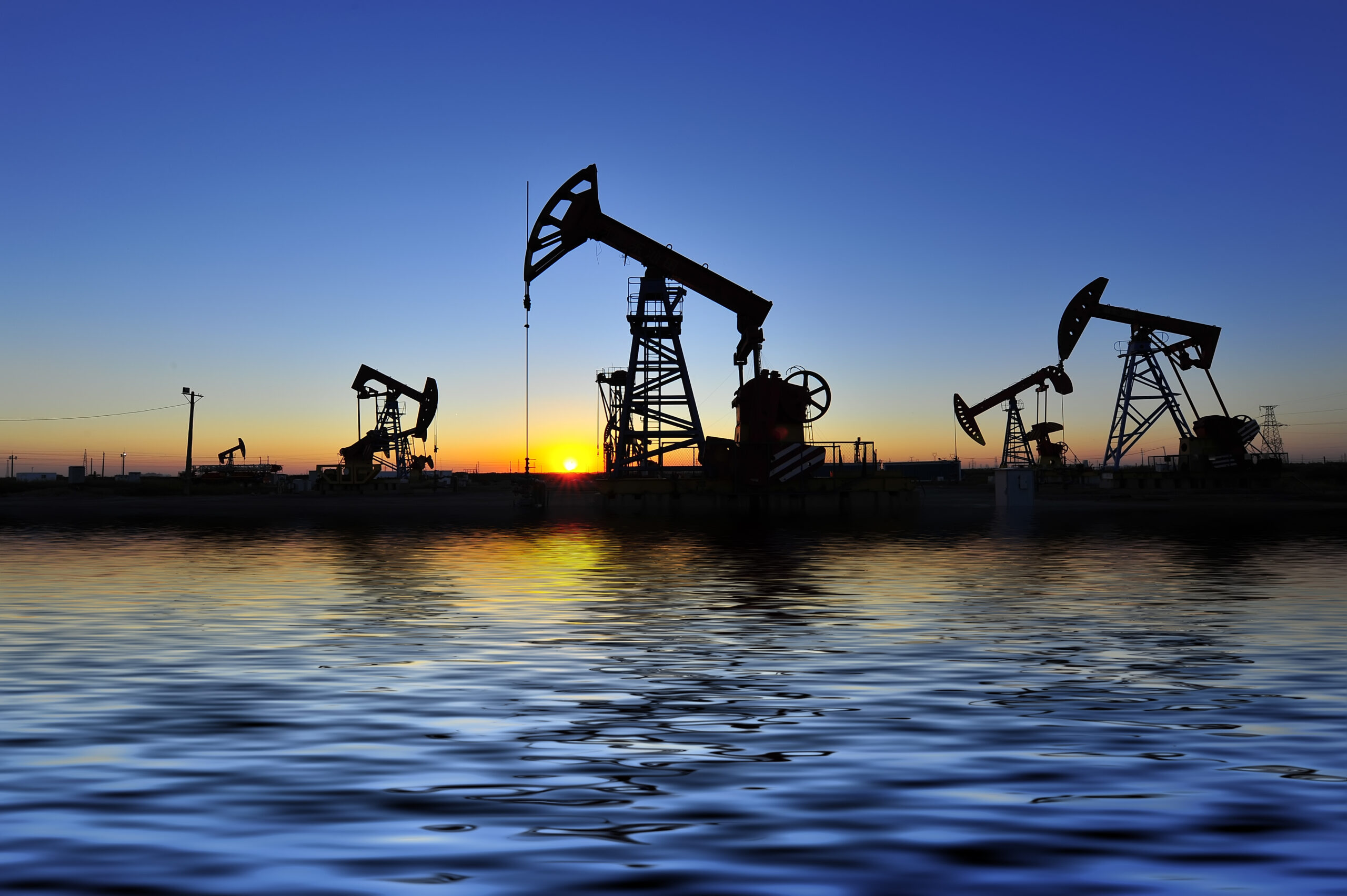
Electrical Submersible Pump (ESP) Up-Time
Challenge
Many oil producing wells require an artificial lift to increase the flow of fluids as the reservoir pressure is not high enough. Typically, electrical submersible pumps (ESPs) are used, but these pumps are not always operating efficiently and can cause costly downtime for operators. Sudden loss of flow can damage the pump, causing lost productivity and expensive pump repair costs. In addition, the lack of sophisticated trip logic can lead to premature trips and loss of production. This problem spans hundreds of pads and thousands of wells.
Solution
To avoid costly downtime operators must be able to detect events where flow drops, indicated by wellhead temperature drop, combined with motor amp and tubing choke data. Historical data and events are used to set up the analytic which is then used to continuously monitor current conditions and detect new emerging problems. Through the use of Seeq’s Asset model Capabilities, minimal effort is required to replicate the analysis automatically across all wells to detect such events.
Benefits
Prior to using Seeq, ESP failure detection and analysis was purely reactive, due to a large number of assets and data per asset. Shifting to proactive management of ESPs can provide oil and gas operators with an annual savings of approximately one million dollars.
Data Sources
- Process Data Historian: OSIsoft PI
- Asset Structure: OSIsoft Asset Framework
Data Cleansing
- “Low-flow” events are disregarded if they occur during well test periods or other maintenance periods, eliminating alert fatigue.
Calculations and Capsules
- Low-flow occurrence identification is a combination of 15 different conditions
- Just one or two conditions alone didn’t give high accuracy for detecting true low-flow events (With 15 layers of context, low-flow detection is accurate)
- Accurate pre-cursor capsules are established as well, consistently predicting when a low-flow event happens in the past and the present
Summarizing Results
- Low-flow occurrences are consistently identified on historical data to give confidence when identified in real-time data
- With accurate low-flow identification, pre-cursor events are also accurately and consistently identified, allowing operations teams to anticipate and avoid costly low-flow events and confidently operate otherwise