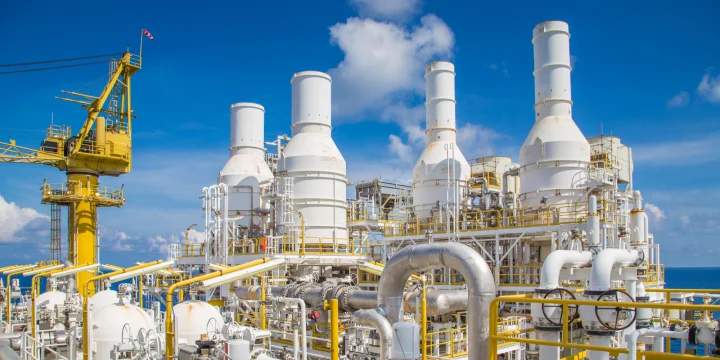
Bearing Failure Prediction
Challenge
At many process manufacturing operations, bearings fail exponentially and with little notice, leading to downtime that can become expensive and making scheduled maintenance difficult. System interdependence often means that a failure of one bearing results in the subsequent failure of other system bearings. Being able to prepare for and prevent the first bearing failure can reduce the costly and harmful effects of unplanned bearing failures.
Solution
Seeq’s advanced analytics application allows SMEs in process manufacturing operations to expertly cleanse data, identify normal operation, calculate statistical thresholds, and identify deviations. The company’s team of data scientists extends the analysis by using the cleansed data and operational context from the SMEs to build a bearing health status signal by running an anomaly detection algorithm. The data scientists finish their work by passing this anomaly detection signal back into Seeq Workbench.
Results
Maintenance is notified of abnormal operations several days before a catastrophic failure. This early warning time enables proactive greasing of bearings to extend the run life, advanced parts ordering, and resource allocation so maintenance cost and downtime are minimized when the failure occurs. For a critical refrigeration compressor, preventing a single bearing failure may result in lost time savings of >$0.5M.
Data Sources
- Process Data Historian like OSIsoft PI or others
- An Asset Framework structure, like OSIsoft PI AF, to quickly scale calculations from one bearing to many
- Supplemental high-frequency data from periodic vendor testing, stored as CSV files uploaded to Seeq
Data Cleansing
Bearing vibration signals were cleansed to remove downtime data
Calculations and Conditions
- Value Search and Formula were used to identify and remove downtime data
- Formula was used to identify “normal operation”—the data set used for calculating the statistical thresholds and the eventual training data set for the Neural Network (NN) model
- Formula was used to calculate significant statistical thresholds based on normal operation period
- Deviation searches were used to identify when one bearing signal deviated from its statistical limit
- Composite condition was used to identify when any bearing signal deviated from its statistical limit
- Seeq Data Lab was used by the Data Science team to pull cleansed, contextualized data from Seeq Workbench and a NN model was used to create a 1-0 anomaly detection signal for each bearing
- Seeq Data Lab was used to scale the analysis from a single bearing to all of the bearings at a particular site
Reporting and Collaboration
- The anomaly detection signals were pushed back to Seeq Workbench where the machinery engineer could use them for his daily machinery monitoring to identify potential bearing failures earlier.
- The results were added into a Seeq Organizer Topic with multiple interactive dashboards for different layers of the Organization.