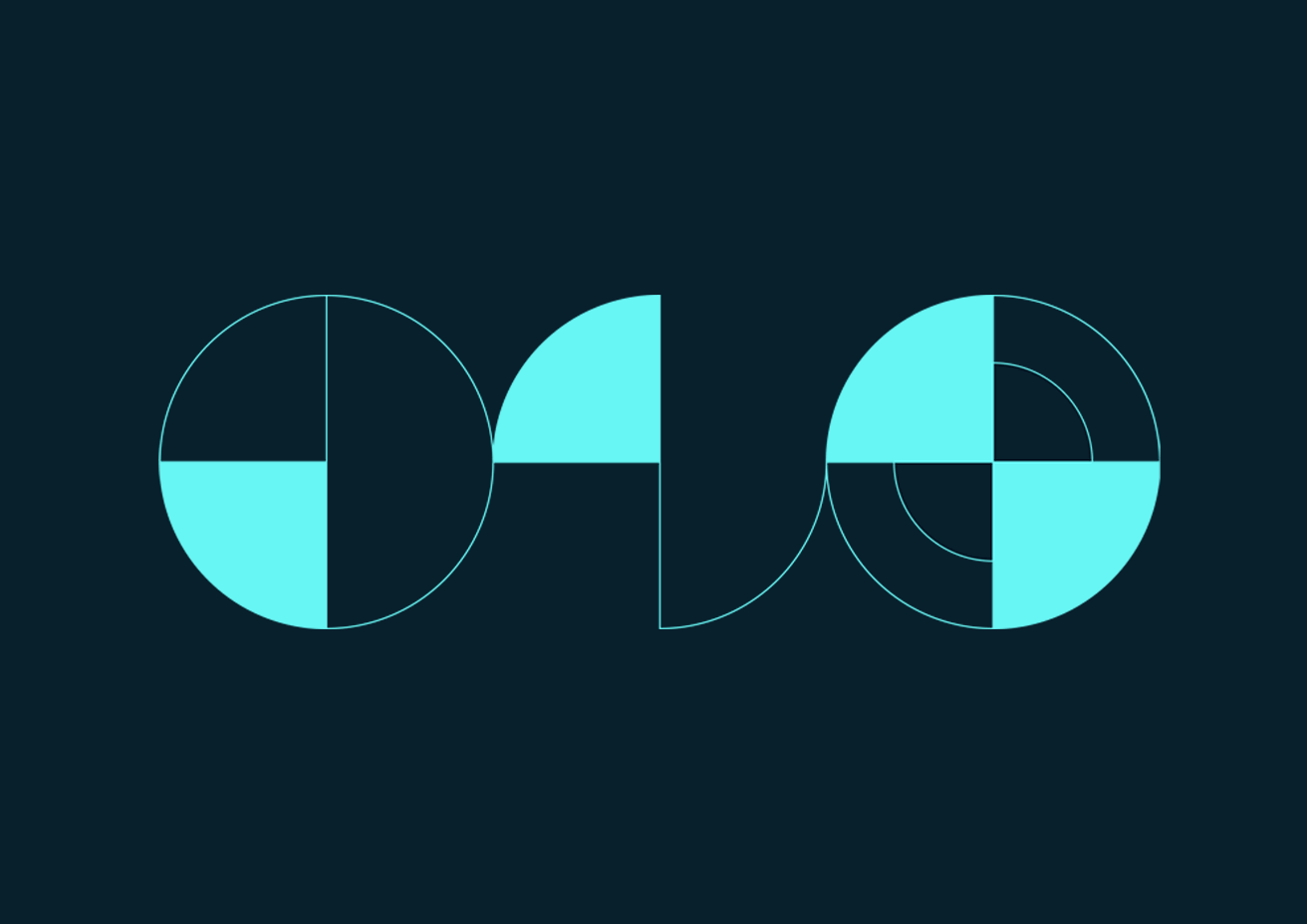
Why is big data mainstream and what exactly does it do?
Back to the Basics of Big Data and Why Companies Are Using It.
Big data is intertwined with analytics: big data is the raw material, and analytics is the process of extracting value or insights from the raw material. Big data has traditionally lead analytics in the sense that data, in particular increasing volumes of data, has been the driving force requiring innovation in ways to extract value from data.
One of the innovators in big data and analytics was Google with their MapReduce algorithm and HDFX file system efforts, both of which were open-sourced in 2004. You may be more familiar with these innovations as “Hadoop,” now an ecosystem of services commercialized by the CloudEra.
Google also developed a feature, “Trends,” which enables users to see the popularity of search terms over time. And if we search on “big data” in Trends we can see it’s increase in popularity over time and what has happened since. Big data searches started an upward climb in May 2011, surpassing searches on machine learning in January 2012. If you’d like to know where the momentum came from, read the McKinsey & Company report “Big data: The next frontier for innovation, competition, and productivity.“ This report, published in May 2011, didn’t cause the flood of big data interest, but it certainly was well timed to market attention.
Since then, “big data” has tapered off in searches, surpassed again by “machine learning” in June of 2017. As information consumers, we experience this in the number of articles about machine learning and artificial intelligence, and the hype and promises from vendors. Which shouldn’t be taken as a sign of big data’s lack of importance. What we also see here is the relationship of data and analytics: first comes the data, then comes the need for insights in that data, in this case machine learning as an approach to gaining insights.
While machine learning may garner more searches at this point, big data isn’t any less important. Industrial companies have been collecting big data—terabytes, petabytes, and zettabytes of sensor data—for decades. For as long as prices have been dropping for hardware, communications networks, storage systems, and processing power (aka, Moore’s Law), the decreasing costs of data generation have challenged then current systems to keep up. And the future holds even more data: the explosion of sensors and pervasive wireless networking systems (aka, IIoT, or Industrial Internet of Things) means big data, for manufacturing markets, is a past, present, and future fact.
The origin, language and use of big data.
Big data, the concept, is as old as computing. The ability to create more data than it was easy to store and use in analytics is simply a fact. This is because the pace of computing has always meant any new computing generation would struggle with innovation in the data generation abilities of new offerings within the time period of its useful life.
Big data, the words, is a well-researched issue. Who used it first, when and how? There is agreement that the first recorded use was by sociologist Charles Tilly, who wrote in a 1980 working paper “none of the big questions has actually yielded to the bludgeoning of the big-data people.” You need to decide for yourself if the usage matches your definition. You can read about the search for the roots of big data in the New York Times, and again, and from Gil Press, a writer for Forbes.
Big data, the definition, is agreed upon: Doug Laney, an analyst with the Meta Group (acquired by Gartner) first used the “3 v’s of big data” (volume, velocity, variety) in a report in February 2001, a decade before the broad recognition and searches for the term described earlier. Of course, some analyst firms like to add their own V words to the equation. Veracity, variability, and value, as three examples.
Finally, big data, as an accepted term, came in June 2013 when the Oxford English Dictionary added it to their list of recognized words.