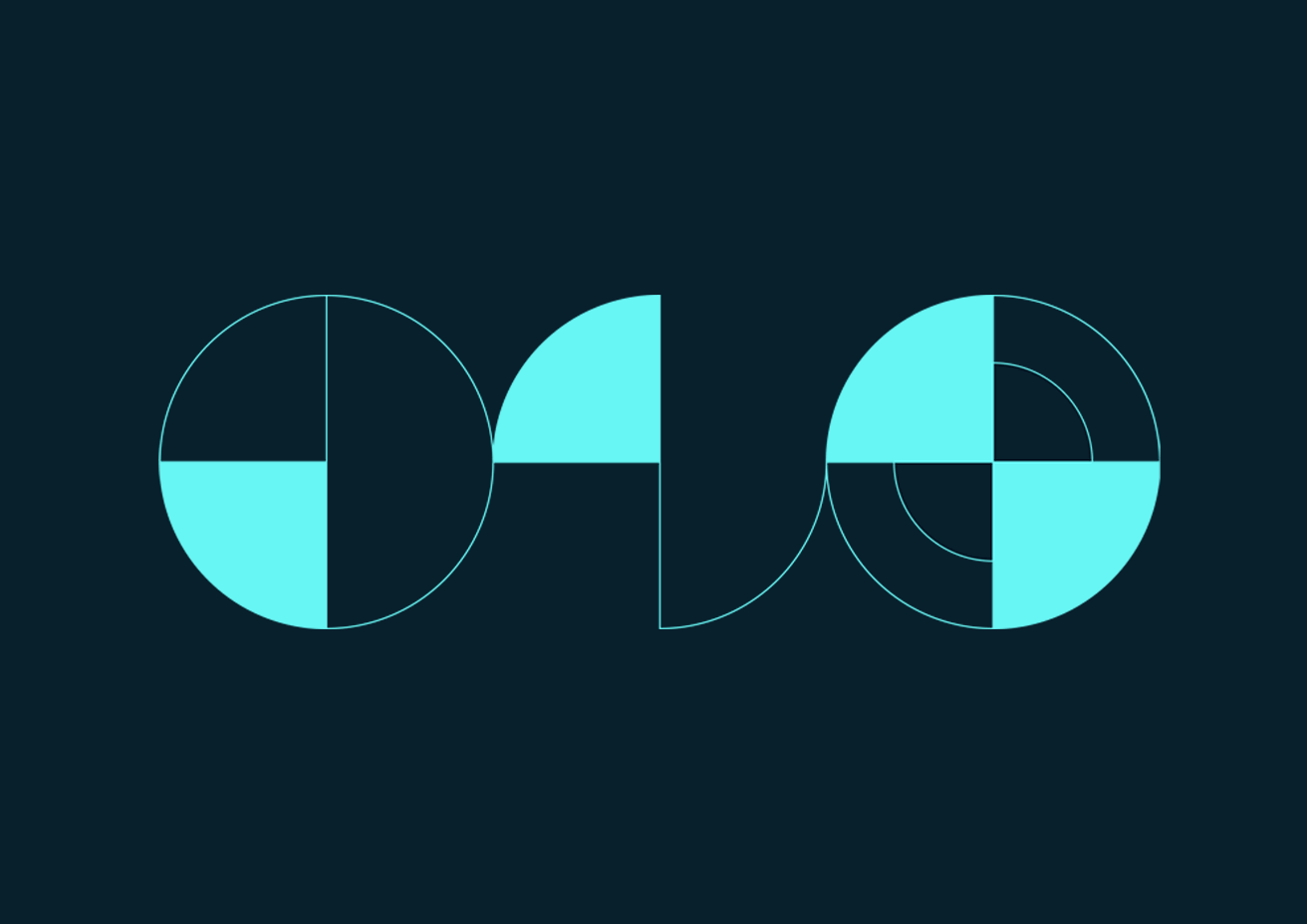
Now is the time to capitalize on your time series data.
Advantages of Using Data Historians and Advanced Analytics Software to Improve Business Outcomes
Seeq continues to expand in deployments and user counts across process manufacturing verticals. These expansions are built on the successful insights achieved by customers using Seeq for predictive, diagnostic, and descriptive analytics. In particular, customers recognize Seeq’s unique understanding of, and features for, working with time series data.
This expertise is hard earned, a testament to the hundreds of years of experience held by Seeq employees, combined with innovations in data science and management which Seeq is leveraging to address customer challenges. Seeq’s handling of interpolation, digital signal processing, and multiple time zones for analytics are just a few examples of the elegance and ease with which Seeq handles the specific challenges of time series data, and the specific requirements of process engineers and subject matter experts working with it.
This has led to questions from customers regarding Seeq offerings that extend beyond ad hoc and self-service advanced analytics. Could Seeq, for example, expand to include support for storing time series data with the same finesse it provides for performing analytics with time series data?
This is typically asked by companies falling into one of three categories: companies that do not currently have a storage solution for their time series data, customers with antiquated time series data storage systems looking for the easiest path to advanced analytics insights, and customers wondering if there is a way to solve two problems at once with modern data storage and modern analytics applications. And when I say “antiquated,” I mean version 2.x historians that are still in operation, or legacy historians that have not had an update for years if not decades.
The answer to the question of Seeq and data storage offerings is no, data storage is not a feature or an area of focus for Seeq. As context, the following points explain the details of Seeq’s priorities and its differentiation from time series data storage offerings.
All hail the historian!
Whatever they are called—process historians, data historians, enterprise historians, process data historians, etc.—historians are an engineering marvel, especially when one considers the design challenges. Historians must support thousands, if not hundreds of thousands or even millions, of small data writes at a variety of sample rates—10K hertz to 1 a day and everything in between—requiring buffering if the system is overloaded, compression for saving space on disk, connectors for hundreds of data sources, and other features.
In addition, the term “system of record” isn’t something historians can just claim, it is earned with a track record for scalability, reliability, and security. And the ecosystem of applications relying on historians for data to enable features like trending and KPI dashboards put historians clearly in the category of a platform offering. The breadth of computer science challenges which historians address may be well solved, but it does not negate the complexity of the problem they address for end users.
Finally, historians have evolved from mere databases for storing signal data. The OSIsoft PI System, as the leading example, is a complete infrastructure solution for organizations, with services for asset modeling, notifications, and other features.
In Seeq, for example, “asset swapping” of analytics is enabled by PI’s Asset Framework. Asset swapping enables Seeq users to take a calculation for one pump and apply it to similar assets for scaling a diagnostic calculation over a portfolio of assets. Another example is PI Notifications which enable Seeq’s predictive analytics to tie into email and other systems to provide early warnings for abnormal operation, asset failure, or required maintenance.
Like the historian, these platform features require a significant investment in computer science and design to solve each particular use case. Seeq leverages these investments, as it does the underlying historian data, which represent thousands of lines of code optimized by years of expertise invested in their design.
Linking Seeq and data historians to improve outcomes
In contrast to the “many small-writes” architecture of historians, and the extended features of historian platforms, Seeq’s architecture is focused on data read performance. Seeq enables process engineers and subject matter experts to find and share insights in stored data to improve production and business outcomes. That means the many-small-writes model of historians is a long way from Seeq’s design point.
In fact, Seeq users often require access to less than 20 signals at once for their analytics. Unfortunately, there isn’t any way to know which signals those are in advance, which requires a close link between Seeq and the historians containing the captured plant data. This should be a point of emphasis for customers: the best practice is to store all signal data in full fidelity because you don’t know what you’ll need at analytics time and summarizing or cleansing the data prior to storage can remove exactly the details needed for insight.
In addition, even if the signal count required for an analysis is not large, for any one signal the user may need thousands to tens of millions of samples to fulfill their requirements (typically between a month to five years of data). This required functionality drives an important design principle of Seeq:
Seeq does not create a copy and summarize or down-sample source data.
Instead, Seeq calculations are based on the actual high-fidelity data, gaps and all. The display of historian data may be simplified to support visualization in Seeq applications, but calculations are on full fidelity data. Converting calculation fidelity to user displays is another example of the specific end user needs Seeq is addressing.
The read-only model, calculation fidelity, and display logic are examples of how advanced analytics software addresses a very different set of requirements from historians. A final example is how Seeq addresses the interactive, high-speed engagement between users and data with a cache, both in memory and on disk, for data required by the user. This works well because there is a high degree of reuse for the data users “request” (the signal they want to analyze over the time period they need).
Caches used by Seeq are not always required depending on historian read performance, but when used may span from megabytes to terabytes in size, depending on the number of users and signals. Based on Seeq’s analysis of user requirements, the Seeq cache is highly optimized for read time, new incoming data, and size. The benefit of this model is fast user access to data to enable interactive analytics at the speed of thought.
A result of Seeq’s approach to enabling analytics is that it can’t go on forever without resources to power both the cache (data size) and the calculations. Thousands of users in Seeq customer deployments require more than a bigger server for analytics, which means software solutions that provide elastic, or on demand, capacity for compute-heavy calculations and queries. Therefore, Seeq is expanding support for customers who need large deployments for their user base, with elastic computing, also known as “scale out,” to enable advanced analytics for a growing number of users.
The combination of historian or data storage solutions, and the specific challenges they address, and Seeq advanced analytics is the framework for the success of Seeq’s growth in the number of end user deployments. Serving as a system of record for process data, historians and time series storage systems capture the breadth and fidelity of the sensor data within a plant. Seeq then enables the separate and complimentary task of user access to any signal for analytics for finding and sharing relevant insights to improve process and business outcomes.
Seeq’s unique and specialized role within this ecosystem will keep us busy with user requirements and new use cases for years to come, well outside of the domain expertise and offerings of historian platforms.