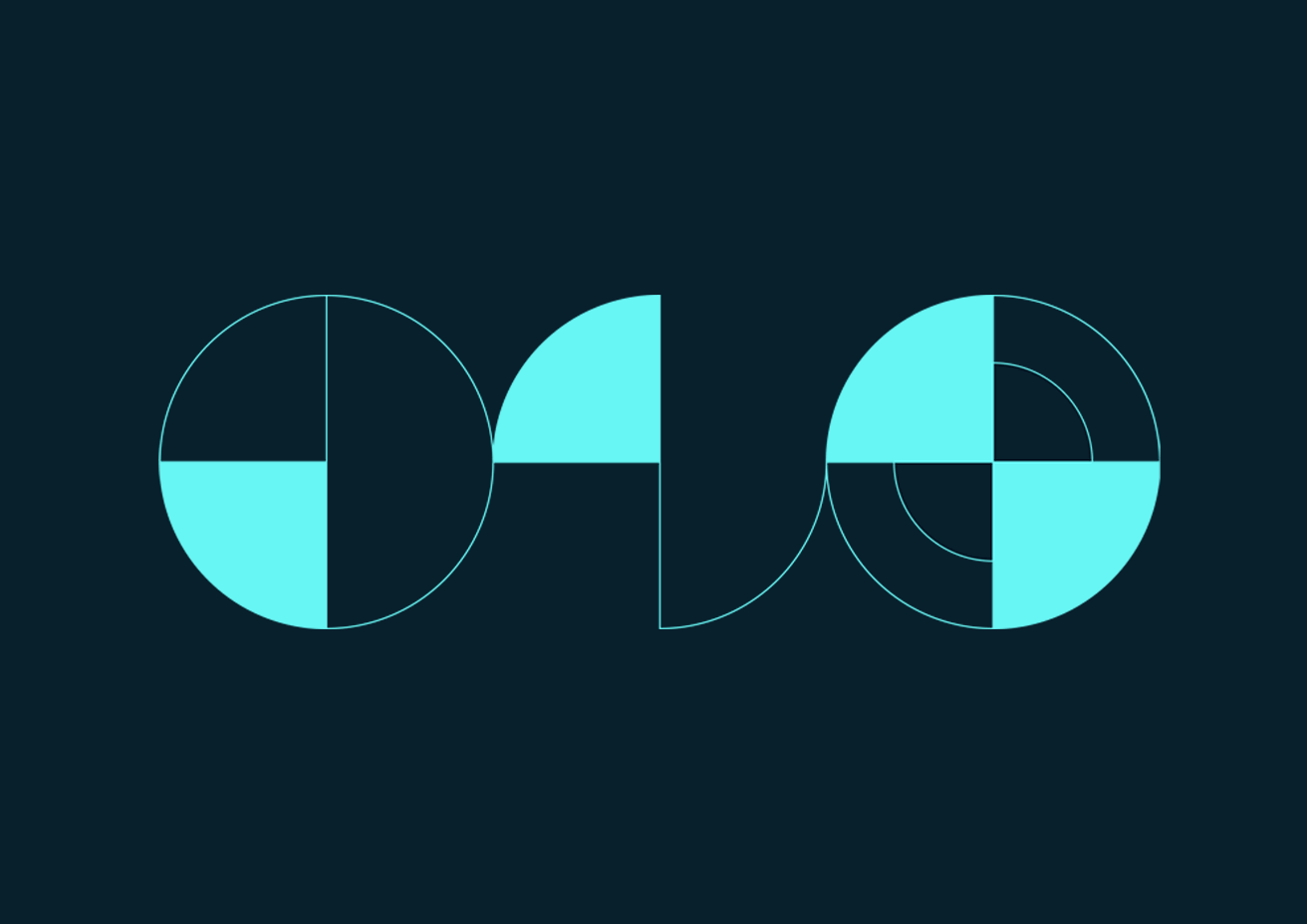
In Search of the ‘Perfect Batch’?
How to Use Advanced Analytics to Meet Strict Quality Standards
Batch process manufacturers in industries like pharmaceutical and food/beverage know it is crucial to maintain tight control of processes so batches meet quality standards. Deviation from specifications is a recipe for disaster.
But the world isn’t perfect, and variability can creep in. So, process engineers pore over historical data to create process parameter profiles, with tolerances, to serve as guides for reducing process variability and increasing yield.
Unfortunately, this approach doesn’t always work. Out-of-tolerance events occur, despite applying the greatest diligence in controlling the Critical Process Parameters (CPPs) of a recipe, as measured by a group of Critical Quality Attributes (CQAs). Often, it becomes clear the number of variables and the cause-and-effect relationships connecting CPPs and CQAs are more complex than realized.
Revealing the ‘perfect batch’
When this happens, successful batch production means acquiring and analyzing detailed production data from historians and other data repositories. This makes it possible to identify “perfect batches,” including all of the associated data. Engineers can then review this information to gain actionable insights.
To do this, engineers have traditionally manually extracted data from an industrial control system or historian to create graphs in Excel. This method can generate some answers, but there are limits on how a modest spreadsheet can be used to understand complex process variability.
Advanced analytics to the rescue
Today, there is an option that goes beyond spreadsheets, offering contextual insights in the moment without requiring assistance from a data scientist to use. Applications focused on advanced analytics, such as Seeq, are a viable alternative that offer robust functionality within a simplified infrastructure. Seeq offers a better mechanism to aggregate and analyze data than spreadsheets, revealing greater insights and intelligence.
Batch process manufacturers no longer need cumbersome tools and techniques such as spreadsheets, data cubes, and data warehouses. Seeq, for example, runs on typical office computers, communicating directly with historians to quickly extract data and present results.
Here’s how this works in Seeq.
Assume you’re examining a production process with six CPPs connected to a unit procedure. Using historical data from perfect batches with acceptable specs on all CQAs, you can now simply and easily graph these six variables from all the previous unit procedures. Curves representing performance from historical CPPs can then be superimposed on top of each other using identical scales to reveal new insights (see the figure below).
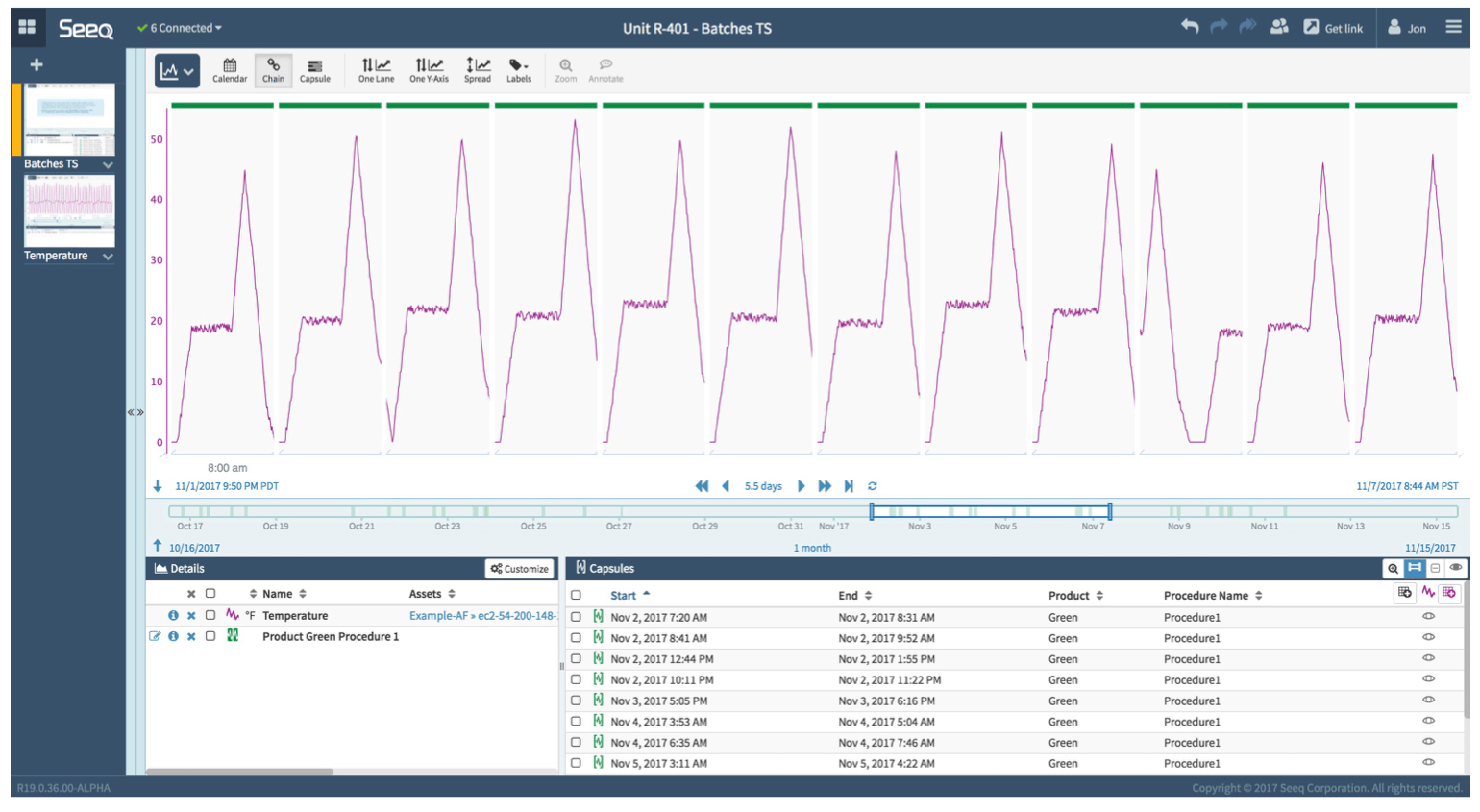
It is easy to see if the curves tend to form a tight group, or if they are spread out, showing different values at various times. Seeq can easily aggregate these curves without the need for complex formulas or macros to establish an ideal profile for each CPP. Engineers can replicate this procedure, resulting in an updated reference profile and boundary for every variable. In the end, this process reveals new opportunities for process optimization.
People bring it together
Despite how advanced analytics have become, they still can’t suggest hypotheses, establish tests, or draw conclusions. We still need process experts and engineers to examine the data, identify trends, and advance theories of what might be happening with out-of-spec CPPs. Human expertise, combined with advanced analytics applications, is needed to test theories and derive cause and effect.
We will always need process engineers and experts to identify cause and effect, advance theories, and interpret conclusions to improve batch manufacturing performance. The key is to put an advanced analytics application like Seeq in the hands of those who understand the process best.