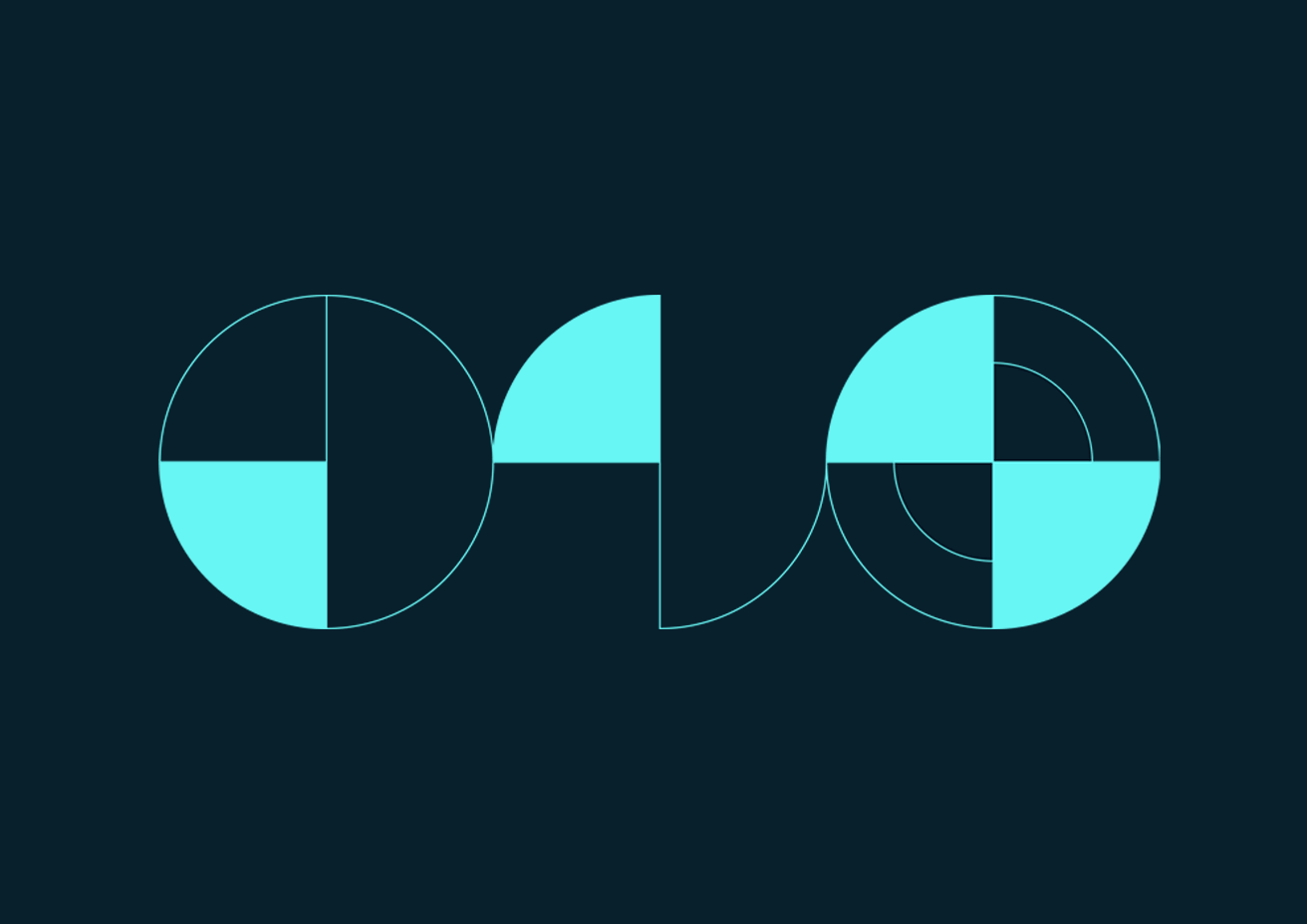
Better Together: Why Connecting IT/OT with Seeq Enables Machine Learning Success
How to Capitalize on Machine Learning Insights for Better, Faster Decision Making
Machine Learning (ML) is a top priority for most manufacturing organizations— and for good reason. Machine Learning models can simulate processes and predict outcomes, drive continuous improvement cycles, and enable manufacturers to proactively maintain and optimize equipment. That’s a big deal; it can mean millions of dollars in savings and efficiencies in a short amount of time.
However, leveraging Machine Learning in manufacturing settings is not as simple as finding the right algorithm or hiring data scientists. The engineers and plant operations personnel that understand their processes and the associated sensor data are critical participants in any effort to apply ML in an industrial context. They’re the folks that must understand and trust the predictions. They will want to carefully vet changes and then verify results.
In these scenarios, Information Technology (IT) personnel like sysadmins and data scientists bring the algorithms and software knowledge – often including programming skills. Operational Technology (OT) employees like chemical engineers and operations managers bring the real-world manufacturing and process knowledge. The last necessary piece is an environment where they can collaborate effectively. Otherwise, promising ML initiatives can end up as frustrating failures.
Seeq provides key components to enable IT/OT teamwork for successful Machine Learning efforts: data access, cleansing and contextualization; algorithm development and iterative workflow; collaboration and knowledge capture; publishing of Machine Learning models with simple point-and-click, workflow-centric interfaces for the OT personnel; and now a rich ecosystem of community-based ML solutions for manufacturing environments via the Seeq Open Source Gallery.
The IT/OT Hurdle
The divide between OT and IT departments is nothing new. Data is often trapped in OT data silos that are difficult for IT employees to access easily. At the same time, OT data may be rich for use with ML, but plant and central group engineers often lack expertise in Machine Learning algorithms. These users tend to gravitate toward “no-code” tools or solutions with intuitive interfaces that plug into existing workflows.
Data scientists, on the other hand, are more comfortable with coding and algorithm development but are relatively new in the manufacturing space. They often lack domain expertise and are using algorithms against data they don’t fully understand. Process data is dirty and usually lacks context, which ultimately leads to inaccurate predictions.
Both sides need each other. Data science teams often feel that models are difficult to explain to engineers and operators, but the lack of collaboration and domain knowledge causes plant employees to distrust the models. Even if data science outputs are accurate, engineers are often skeptical because they must be able to explain results and any associated actions to operators, managers, and other stakeholders. Tools developed by data science groups can therefore get stuck in prototyping because they lack the buy-in needed to move forward.
Why Seeq is Essential
Seeq integrates the IT and OT worlds to give Machine Learning initiatives a much higher success rate for manufacturing organizations. Seeq Workbench, Seeq Organizer and Seeq Data Lab plug into both the established OT world of process management and the rich emerging world of data science and cloud technologies on the IT side.
First, Seeq connects to all underlying data sources, including historians, MES, LIMS, maintenance systems, or any other data source. This combination of relevant data enables a rich contextual foundation from which to perform analyses.
Second, Seeq’s purpose-built calculation engine for industrial data addresses a key problem: data preparation. Everyone knows the “garbage in/garbage out” axiom, but data scientists may be unable to determine if data is bad due to lack of operations knowledge. In addition, data can be relevant one minute and outdated the next. Knowledge capture from engineers who understand the plant is critical to ensuring the integrity and relevance of the data being modeled. Seeq enables engineers to access the data (as signals), cleanse and contextualize it (with conditions) and then collaborate in a variety of ways with data scientists to ensure optimal outcomes – via Seeq’s sharable workbooks, worksheets, journals, topics and Jupyter notebooks.
Finally, Seeq works seamlessly with Azure Machine Learning Studio, AWS SageMaker, Anaconda, and the integrated Python environment in Seeq Data Lab. Data scientists can develop and use their algorithms using the tools they know and love, then publish their models to their OT audience as Seeq Add-ons. This enables an iterative set of experiments between the data scientist and engineer. The plausibility and the accuracy of the predictions and improves over time. Rapport and trust are built, and both sides come to rely upon the other for success at their jobs.
Driving Better Outcomes
Building on Seeq’s efforts to date, Seeq is working to drive increased ML value at an industry level. Companies can deploy their own proprietary algorithms, and they are also free to draw upon the growing ecosystem of Seeq-developed, third-party and open-source options. For example, Seeq and the broader community are now actively publishing new algorithms and Add-ons via the Open Source Gallery.
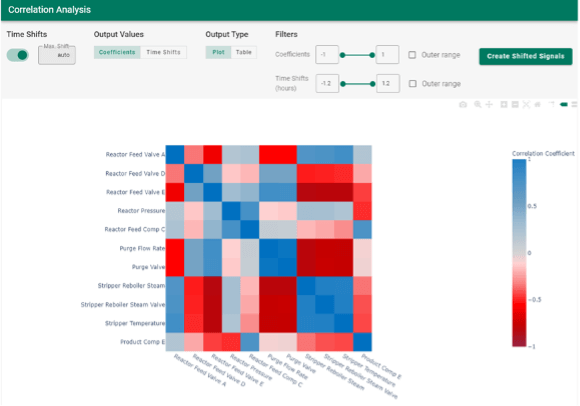
This level of collaboration among experts opens new opportunities to overcome common challenges and needs. For example, all petrochemical companies must meet EPA emissions standards. By giving these companies access to off-the-shelf algorithms that are easy to deploy while still having the flexibility to maintain confidentiality for proprietary calculations and measurements, Seeq is helping to solve industry-level challenges. With Seeq’s collaborative capabilities, expertise and algorithms can come from anywhere.
A few examples may help to illustrate the potential:
- A large oil and gas company uses a Seeq-developed solution to monitor a critical piece of equipment. Operations teams use Seeq to understand the operating ranges that improve performance output while maximizing the lifetime of the asset. Seeq also enabled the data science team to operationalize and distribute an internally developed solution for equipment monitoring and what-if analysis.
- The data science team in a pharmaceutical company used Seeq to operationalize their solution for detecting sensor drifts. Seeq enables their manufacturing engineers to collaborate with the data science team. This two-way interaction builds trust and helps sustain the internally developed solution.
Collaboration is Key
Seeq’s integrated approach to Machine Learning enables operations and data science teams to work together to optimize equipment and processes. By making all sources of data accessible from anywhere, allowing users to cleanse and contextualize information, and enabling real time collaboration between IT and OT, Seeq’s flexible approach to Machine Learning capitalizes on users’ strengths and brings teams together to optimize operations outcomes.
Download this on-demand webinar from Seeq’s resident machine learning experts to learn how you can bridge data science initiatives and SMEs with Seeq’s expanding ML features.
Schedule a demo of Seeq’s leading advanced analytics application today!