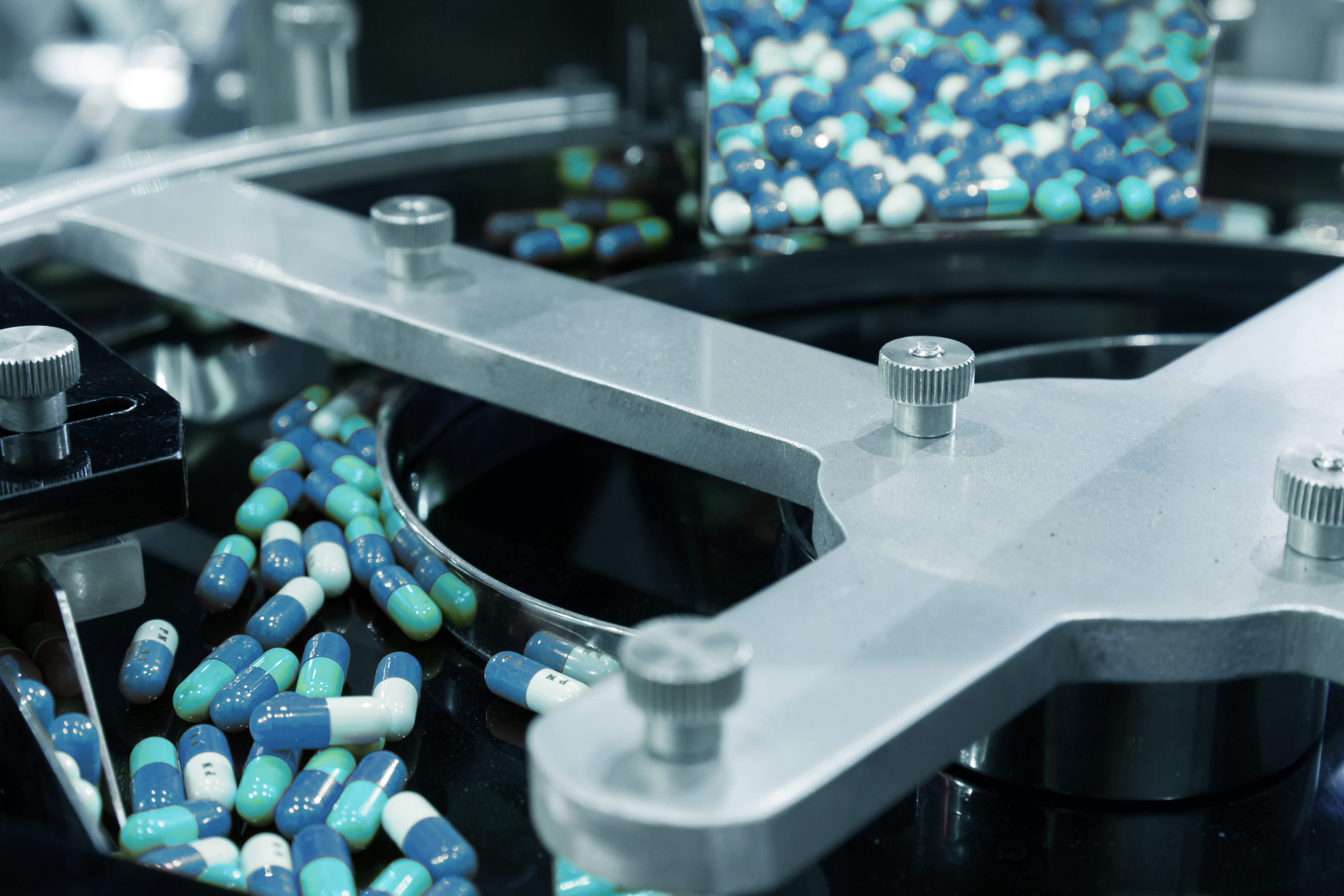
Continuous Drug Product Quality by Design (QbD) Modeling
Challenge
During the manufacture of pharmaceuticals, it is critically important to maintain certain process parameters to ensure the batch meets stringent quality requirements. Critical parameters (such as temperature or agitation) have a required range. The batch will fail if the batch was not in range on each measure, causing millions of dollars of rework and lost revenue.
Now, a new approach enables drug makers to change the definition of the process parameters that define a quality batch. The Quality by Design (QbD) approach considers multiple variables and their interactions.This utilizes experimental models to broaden the range of a single variable if all other parameters are within range. For example, a temperature deviation of five degrees might be acceptable for quality purposes if the other variables are within specified ranges.
QbD is an improvement because it expands the process design space to reduce the number of major or critical deviations, which can run into the tens of thousands of dollars for major pharmaceutical companies.
As a pharmaceutical is commercialized, the process is transferred from research and development (R&D) to a commercial production plant. Typically, this results in the loss of much information around the design due to differing equipment and personnel. A major pharmaceutical manufacturer needed to improve the QbD modeling process it used in R&D, enabling it to avoid failed batches and deviations in production. It also wanted to maintain the transfer of its QbD information moving from R&D to production.
Solution
Using Seeq, the pharmaceutical company was able to analyze a continuous pharmaceutical drug product wet granulation step with a Design of Experiments (DOE) to determine a multivariate QbD process model. The goal was to apply the multivariate design space to commercial production for process monitoring and identification of deviations.
The experimental data was time warped to align the signals based on their sensor location in the continuous process. The data was then cleansed to model only steady-state operation. The design space model was then built using Seeq’s prediction tool for critical quality attributes (CQAs) and applied to commercial production to show a multivariate boundary for continuous process monitoring.
Results
Using Seeq to bridge the gap between data historians and organizational groups, the customer was able to transfer the complete process design information for process monitoring in commercial production. This resulted in an expanded multivariate design space with minimized quality deviations, a major potential cost savings. The customer utilized the online multivariate design space model to automatically flag quality deviations with capsules in Seeq.
Data Sources
- Microsoft SQL: Laboratory results
- OSIsoft PI: Process data
Data Cleansing
- Filtered experimental data to steady-state period for modeling
Calculations and Conditions
- Time warp of signals to align based on sensor location in process
- Developed prediction model for critical quality attributes (CQAs)
- Applied design space as boundaries for commercial production
Reporting and Collaboration
- R&D process design information used as process monitoring in commercial production
- Deviations from design space boundaries flagged with capsules
- Minimized out-of-specification product by expanding to a multivariate design space